A New Definition of Agency: Principles for Modelling AI Agents and Their Incentives
Artificial intelligence (AI) is rapidly becoming an integral part of our daily lives, from personalized recommendations on social media to autonomous driving cars. As AI systems become more advanced and ubiquitous, ensuring their alignment with human values and goals becomes increasingly important. This requires understanding the incentives that AI agents face and how they make decisions.
To address this challenge, a group of researchers has proposed a new formal definition of agency that provides clear principles for modelling AI agents and their incentives. In this article, we will explore this new definition and its implications for the development of safe and aligned AI.
What is Agency?
In the context of AI, agency refers to the ability of an agent to act autonomously in pursuit of its goals. An agent can be a robot, an intelligent software system, or even a human. The key feature of agency is the ability to make decisions based on a set of internal goals or objectives.
The concept of agency is fundamental to AI research because it allows us to model the behavior of intelligent agents in different scenarios. By understanding the incentives that an agent faces, we can predict its behavior and design systems that align with human values and goals.
The New Definition of Agency
The new definition of agency proposed by the researchers is based on the concept of causal influence diagrams (CIDs). CIDs are a graphical representation of decision-making situations that allow us to reason about agent incentives.
The new definition of agency can be stated as follows:
An agent is a causal influence diagram (CID) that has the following features:
- The CID has a set of internal nodes that represent the agent’s goals or objectives.
- The CID has a set of external nodes that represent the agent’s environment or the world in which it operates.
- The CID has a set of decision nodes that represent the agent’s actions.
- The CID has a set of utility nodes that represent the agent’s preferences or values.
This definition provides a formal framework for modelling AI agents and their incentives. By using CIDs, we can represent the goals and preferences of an agent in a clear and concise way, making it easier to reason about its behavior.
Implications for AI Safety
The new definition of agency has important implications for the development of safe and aligned AI systems. By understanding the incentives that an AI agent faces, we can design systems that align with human values and goals. For example, if we want an AI system to act in a way that is beneficial to humans, we need to ensure that its internal goals are aligned with human values.
One potential application of the new definition of agency is in the development of value-aligned AI systems. These are AI systems that are designed to pursue human values and goals in a way that is safe and beneficial. By modelling the incentives and decision-making processes of AI agents using CIDs, we can ensure that these systems act in accordance with human values and goals.
Another potential application is in the development of AI safety mechanisms. By understanding the incentives and decision-making processes of AI agents, we can design safety mechanisms that prevent them from taking actions that are harmful or undesirable. For example, we can use constraints or penalties to discourage an AI agent from taking actions that are harmful to humans.
Conclusion
The new definition of agency proposed by the researchers provides a formal framework for modelling AI agents and their incentives. By using causal influence diagrams, we can represent the goals and preferences of AI agents in a clear and concise way, making it easier to reason about their behavior. This has important implications for the development of safe and aligned AI systems, as it allows us to ensure that these systems act in accordance with human values
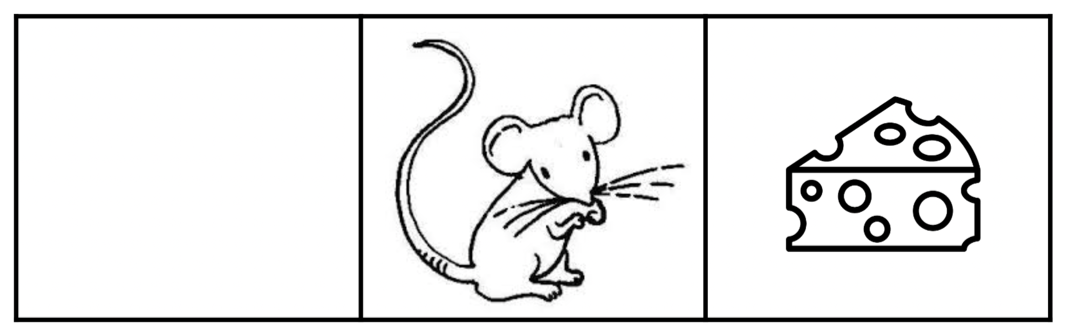
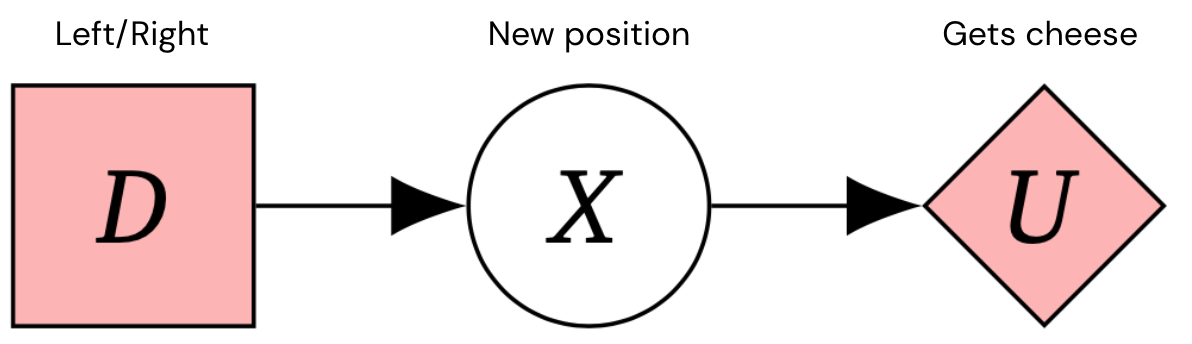
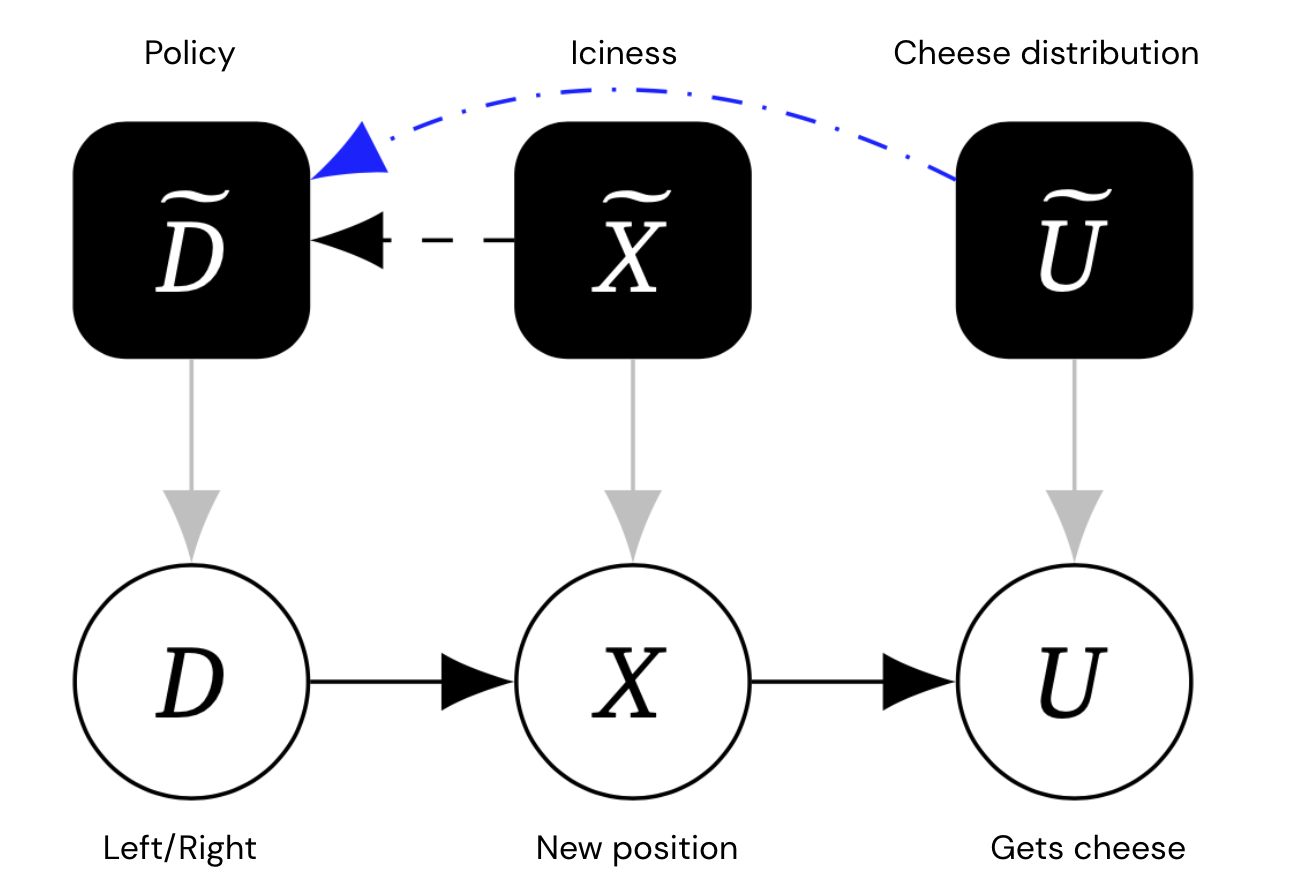
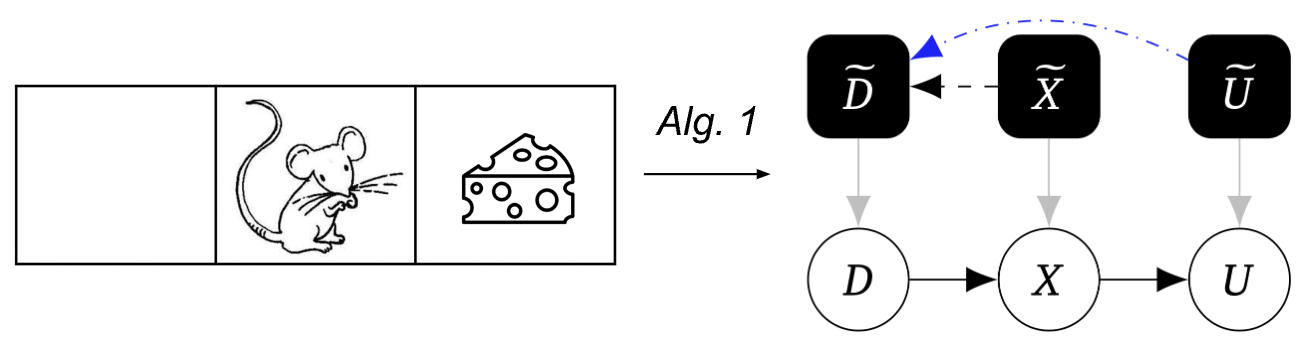
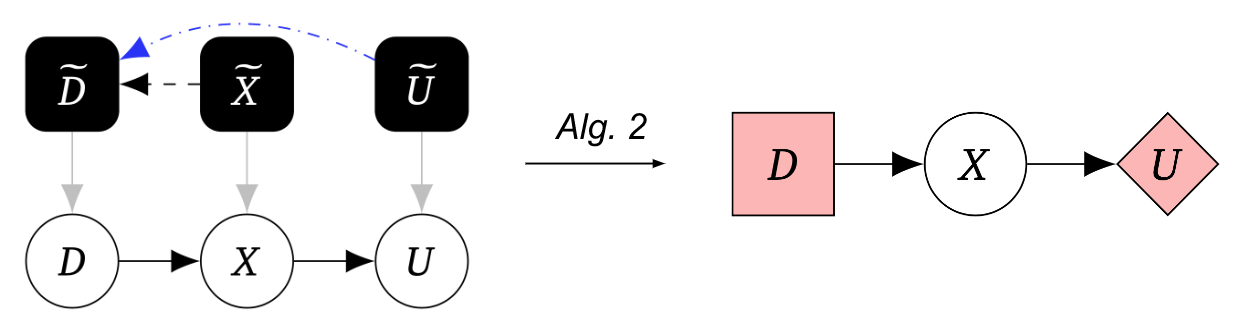
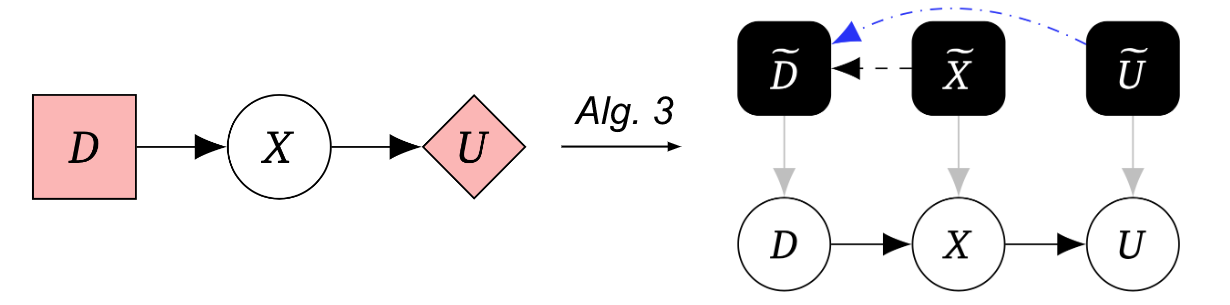
Better safety tools to model AI agents
We proposed the first formal causal definition of agents. Grounded in causal discovery, our key insight is that agents are systems that adapt their behaviour in response to changes in how their actions influence the world. Indeed, our Algorithms 1 and 2 describe a precise experimental process that can help assess whether a system contains an agent.
Interest in causal modelling of AI systems is rapidly growing, and our research grounds this modelling in causal discovery experiments. Our paper demonstrates the potential of our approach by improving the safety analysis of several example AI systems and shows that causality is a useful framework for discovering whether there is an agent in a system – a key concern for assessing risks from AGI.
Author – Murari
Excited to learn more? Check out our paper. Feedback and comments are most welcome. Play sprunki phase 28 Anytime, Anywhere!